The rapid advancements in artificial intelligence (AI) and machine learning (ML) are fundamentally transforming the way we approach health, fitness, and wellness. These technologies are not only making personalized health solutions more accessible but are also revolutionizing the way individuals track and optimize their physical and mental well-being. With an increasingly data-driven world, AI and ML offer innovative ways to understand, monitor, and manage our health, tailoring fitness programs, nutrition plans, and wellness practices to each individual’s unique needs and goals.
This article delves into how AI and ML are shaping the future of personalized health, particularly through apps and tools like Noom, Fitbit, and MyFitnessPal. These platforms leverage data-driven insights to provide highly customized recommendations, optimizing fitness, nutrition, and overall wellness based on a person’s behavior, preferences, and health data.
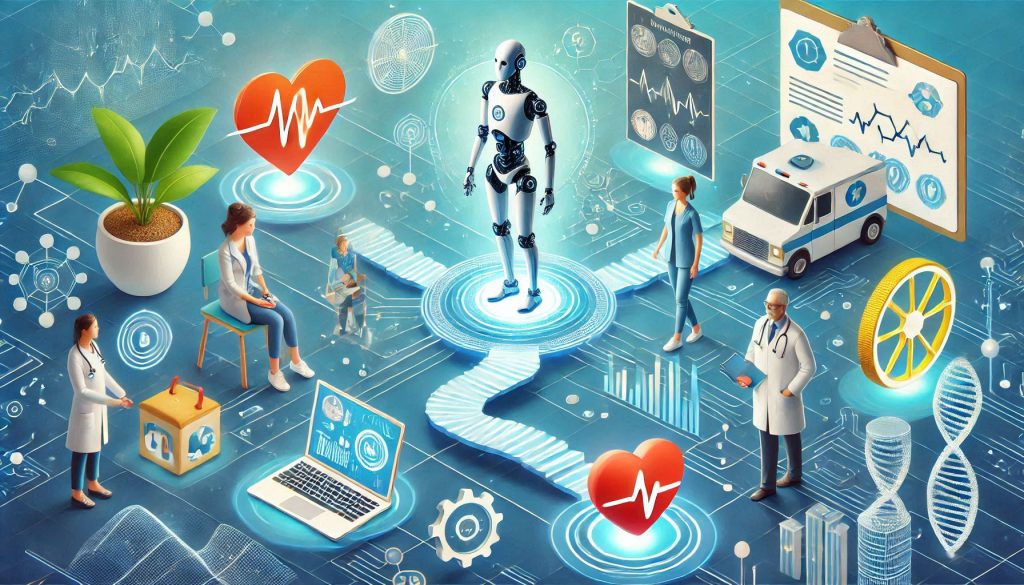
Artificial Intelligence and Machine Learning: What They Are
To understand their impact on personalized health, it’s essential to first define what artificial intelligence and machine learning are and how they work.
Artificial Intelligence (AI) refers to the simulation of human intelligence in machines programmed to perform tasks that typically require human cognition, such as decision-making, problem-solving, and pattern recognition. AI algorithms can process vast amounts of data, identify patterns, and make predictions or recommendations based on that data.
Machine Learning (ML) is a subset of AI that enables machines to learn from data without being explicitly programmed. ML algorithms can analyze and interpret data, improving their accuracy over time. ML’s ability to adapt and learn from new information makes it particularly effective for personalized health solutions.
These technologies can analyze various forms of data—whether that’s data on physical activity, nutrition, sleep, heart rate, or even genetic predispositions—to deliver tailored health recommendations. By using AI and ML, personalized health systems can anticipate needs and continuously adapt to provide the best possible outcomes for individuals.
Personalized Health: A Brief Overview
Personalized health refers to an approach that tailors health interventions to individual characteristics, needs, and preferences. Traditionally, healthcare and wellness strategies were designed based on a “one-size-fits-all” model. However, this approach often failed to address the nuances of individual health needs.
With the advent of AI and ML, the focus has shifted toward creating health strategies that are highly specific to the individual. Whether in the form of tailored fitness routines, nutrition plans, mental wellness programs, or chronic disease management, personalized health allows individuals to achieve better outcomes by aligning health strategies with their genetic, biological, and behavioral data.
The three main areas where AI and ML are playing a crucial role in personalized health are fitness, nutrition, and wellness. Let’s explore how these technologies are transforming each of these domains.
Revolutionizing Fitness with AI and Machine Learning
Fitness has traditionally been a domain that relies heavily on generic advice—whether through workout classes, fitness videos, or one-size-fits-all gym plans. However, AI and ML are changing the way we approach exercise by personalizing fitness plans based on individual data. Apps and devices like Fitbit, Peloton, and Freeletics have pioneered the use of AI and ML in fitness, bringing a data-driven, personalized approach to physical activity.
Fitness Tracking and Personalized Workouts
Wearable devices such as Fitbit, Garmin, WHOOP, and Oura Ring are equipped with sensors that track key metrics like heart rate, steps, calories burned, and even sleep patterns. By collecting continuous streams of data, these devices provide insights into an individual’s activity level, recovery, and overall health status. AI and ML algorithms analyze this data to deliver personalized workout plans and health recommendations.
For example, Fitbit uses AI to create a fitness profile for each user, adapting to their specific fitness levels and goals. It can recommend exercises based on a person’s fitness data, adjusting over time to ensure that workouts are appropriately challenging. Additionally, these devices can track progress and provide feedback, ensuring that users are moving toward their fitness goals at the optimal pace.
Predictive Analytics for Injury Prevention
AI and ML are also making strides in injury prevention. By analyzing patterns in an individual’s movements and exercise data, AI can predict when a person might be at risk for injury. For instance, wearable devices can track gait, posture, and recovery time, using this data to flag potential risks. With this knowledge, users can adjust their routines, make necessary changes to form, or seek medical advice before a minor strain turns into a more severe injury.
Virtual Personal Trainers
AI-powered virtual trainers, such as those found on platforms like Freeletics, Aaptiv, and Peloton, provide highly personalized training experiences. These virtual coaches can adapt workouts based on a user’s fitness level, progress, and preferences. They use ML algorithms to continuously refine recommendations, ensuring that workouts remain engaging and challenging.
By incorporating AI, these platforms can analyze user performance and offer real-time feedback during workouts. If a person is struggling with a particular exercise or not meeting their target heart rate, the AI can adjust the workout in real-time to keep them on track. This approach allows for a level of personalization that traditional fitness coaches may struggle to provide, especially when working with a diverse group of clients.
Transforming Nutrition with AI and Machine Learning
Nutrition is another area where AI and ML are having a transformative impact. In the past, meal planning and diet management were often based on general guidelines or rigid diets. Today, AI and ML are enabling customized nutrition plans based on individual needs, preferences, and health conditions. Apps like MyFitnessPal, Noom, and PlateJoy are at the forefront of using AI to provide personalized nutrition recommendations.
Personalized Meal Plans and Diets
AI-powered apps can analyze individual dietary preferences, health goals, and even genetic factors to suggest personalized meal plans. For example, Noom uses behavioral science along with AI to help users make sustainable, healthy food choices. By tracking food intake, monitoring physical activity, and offering support through personalized coaching, Noom tailors its approach to each user’s unique journey.
Similarly, apps like MyFitnessPal allow users to log their meals and track nutrient intake, using AI to offer feedback and adjustments. Over time, the app learns a user’s eating habits and can make increasingly personalized recommendations. By analyzing a user’s data, these apps can also recommend food choices that align with specific health goals, whether that’s weight loss, muscle gain, or improving overall health.
AI in Nutritional Supplements
AI is also being integrated into personalized supplementation strategies. Companies like Care/of and Ritual leverage AI to create personalized vitamin and supplement plans based on individual health data, lifestyle factors, and even genetic testing. By analyzing a person’s lifestyle, diet, and health status, AI can recommend the most beneficial supplements to support wellness goals. This is an important development, as personalized supplementation is proving to be more effective than taking generic supplements based on broad recommendations.
Nutrigenomics: AI in Gene-Based Nutrition
AI and ML are being increasingly used to analyze genetic data to create highly tailored nutrition plans. Nutrigenomics is the study of how our genes influence our response to food, and AI plays a crucial role in analyzing this genetic data to recommend diet changes that align with individual genetic makeup. Companies like DNAfit and 23andMe are integrating AI to offer insights on how a person’s genetic profile affects nutrient absorption, food sensitivities, and metabolic responses.
By using these AI-powered insights, individuals can make smarter food choices that optimize their health and wellness based on their unique genetic blueprint.
Wellness and Mental Health: AI-Powered Solutions for Holistic Health
AI and ML are also playing a significant role in improving mental wellness by offering personalized mental health strategies. Moodfit, Calm, Headspace, and Woebot are just a few examples of mental health apps that leverage AI to support emotional well-being.
Mental Health Monitoring
Mental health apps can monitor users’ moods, behaviors, and stress levels through data inputs such as mood journaling, activity tracking, and sleep patterns. AI analyzes this data to provide personalized recommendations for improving mental health. For example, if the app detects that a user is stressed based on changes in sleep patterns or physical activity, it might recommend specific exercises, meditations, or stress management techniques tailored to the individual.
Personalized Therapy
AI-powered chatbots like Woebot offer users an interactive, personalized therapeutic experience. These bots use natural language processing (NLP) to understand the user’s emotions and thoughts, providing tailored cognitive behavioral therapy (CBT) exercises. Woebot is designed to learn from its conversations with users, adapting its responses based on the individual’s needs and progress.
These tools make mental health support more accessible, offering real-time, personalized interventions at any time of day or night.
Challenges and the Future of Personalized Health with AI and Machine Learning
The integration of Artificial Intelligence (AI) and Machine Learning (ML) into personalized health is transforming the way we approach fitness, nutrition, and overall wellness. These technologies have immense potential to create highly tailored healthcare solutions, offering individuals more precise, effective, and accessible ways to optimize their well-being. However, despite their promise, the journey to fully realizing personalized health powered by AI and ML comes with a series of challenges that need to be addressed before these technologies can achieve their full potential.
In this article, we will explore the key challenges hindering the widespread adoption of AI and ML in personalized health, as well as look toward the future of these technologies in transforming the health and wellness landscape.
Current Landscape: AI and ML in Personalized Health
Before diving into the challenges, it’s essential to understand the promise AI and ML hold for personalized health. AI algorithms and ML models are designed to analyze massive amounts of data, recognize patterns, and offer personalized recommendations based on individual needs and goals. Whether it’s tracking fitness metrics, recommending nutritional plans, or providing real-time mental health support, these tools are transforming healthcare and wellness.
For instance:
- Fitness: Wearables like Fitbit and Oura Ring monitor physical activity, heart rate, sleep patterns, and even recovery, offering users personalized insights and recommendations to improve their health and fitness.
- Nutrition: Apps like MyFitnessPal and Noom help individuals track their food intake, offering tailored meal suggestions and long-term dietary goals.
- Mental Health: AI-driven platforms such as Woebot offer mental health support, using natural language processing (NLP) to provide personalized therapeutic experiences and guidance.
While these tools are already providing meaningful results, we must recognize that several hurdles must be overcome before AI and ML can become a mainstream solution for personalized health.
Key Challenges in Personalized Health with AI and Machine Learning
Data Privacy and Security Concerns
One of the most significant challenges in the adoption of AI and ML in personalized health is the handling of sensitive personal data. Health-related data, including fitness metrics, genetic information, sleep patterns, and mental health assessments, are highly personal and private. AI-powered health apps and wearables collect vast amounts of this data to create personalized insights, but with it comes the responsibility of protecting user privacy.
Challenges:
- Data breaches: Health data is often a target for cyber-attacks. A breach could expose a user’s private health information, leading to financial, emotional, and reputational damage.
- Regulation: There is a need for stricter regulations to govern the use and storage of personal health data to ensure transparency and security. Regulations such as the Health Insurance Portability and Accountability Act (HIPAA) in the U.S. provide guidelines, but new technologies may present gaps in existing frameworks.
Solution:
- Companies need to prioritize data encryption and implement robust security protocols to ensure the safety of user information.
- Clear data usage policies that allow users to understand exactly how their data will be used and shared will also foster trust.
Bias and Inequality in AI Algorithms
Another significant challenge lies in the potential bias embedded in AI and ML algorithms. AI models are trained on large datasets to make predictions or recommendations. However, if these datasets are not diverse enough, the AI may produce biased results that fail to account for individual differences across different demographics, such as race, gender, age, and socio-economic status.
Challenges:
- Data underrepresentation: Many datasets used to train AI models are not diverse, leading to skewed recommendations that might not be effective for all groups.
- Inequitable outcomes: A lack of diversity in training data can result in health recommendations that are not inclusive, leading to disparities in the quality of care and access to personalized health solutions.
Solution:
- The future of AI in personalized health depends on creating diverse, representative datasets that include people from all backgrounds and health conditions.
- Bias correction algorithms and continuous monitoring of AI systems will help ensure fairness and equity in health recommendations.
Accuracy and Reliability of AI Predictions
Although AI and ML systems are designed to learn and improve over time, their accuracy and reliability remain a concern in many areas of health. The data-driven predictions they generate can be incredibly useful, but they are not always 100% accurate, especially when it comes to diagnosing health conditions, making dietary recommendations, or suggesting workouts.
Challenges:
- Unreliable predictions: In some cases, the suggestions or insights generated by AI-powered health tools may be off-mark or inaccurate, leading users to follow advice that might not be optimal.
- Overdependence: Users might rely too heavily on AI recommendations, disregarding traditional methods or professional medical advice that may be more appropriate for their condition.
Solution:
- Continuous validation and improvement of AI models using real-world feedback from users and healthcare professionals will help improve their accuracy.
- AI should be viewed as a complementary tool to traditional healthcare and wellness practices, rather than a complete replacement.
Limited Access to Advanced Technologies
While AI and ML are transforming healthcare in many regions, access to these advanced technologies is still limited in certain parts of the world, particularly in lower-income countries or rural areas. Not all individuals have access to the internet, smartphones, or wearables like Fitbit or Apple Watch, which are essential for leveraging these technologies.
Challenges:
- Digital divide: Socioeconomic disparities mean that access to AI-powered health tools is not equally distributed. Many individuals are excluded from the benefits of personalized health due to lack of access to technology.
- Cost barriers: High-end wearables and AI-based health apps often come with premium pricing, which can be a barrier for people in lower-income brackets.
Solution:
- Affordable, low-cost health tech solutions must be developed to cater to a broader population.
- Governments and organizations can invest in initiatives that provide wider access to digital health technologies, especially in underserved areas.
Integration with Existing Healthcare Systems
For AI and ML to reach their full potential in personalized health, they need to be seamlessly integrated into existing healthcare systems. Currently, many health apps and wearables operate independently of healthcare providers, which can make it difficult for users to share their health data with doctors or receive coordinated care.
Challenges:
- Fragmentation: The lack of integration between AI-powered health tools and traditional healthcare systems limits the ability to provide comprehensive, personalized care.
- Interoperability: Different health apps and devices may not communicate with each other, preventing data from being used effectively across platforms and by healthcare providers.
Solution:
- Developing interoperable platforms that allow seamless sharing of health data between devices, apps, and healthcare providers will improve the effectiveness of personalized health recommendations.
- Partnerships between tech companies, healthcare providers, and insurance companies will help foster collaboration in delivering integrated healthcare services.
The Future of Personalized Health with AI and Machine Learning
Despite these challenges, the future of personalized health with AI and ML is incredibly promising. As technology advances, we can expect significant improvements in the personalization and accessibility of healthcare solutions. Below are a few trends and developments that will shape the future of personalized health.
Advancements in Predictive Analytics and Preventative Care
In the future, AI and ML will become increasingly adept at predicting health conditions before they manifest. By analyzing data from wearables, genetic tests, and even environmental factors, AI can identify early warning signs of conditions like heart disease, diabetes, and mental health issues. Predictive analytics will allow individuals to take proactive measures to prevent these conditions, rather than waiting for symptoms to appear.
AI-Driven Precision Medicine
AI and ML will help revolutionize precision medicine by tailoring treatments to an individual’s genetic makeup, lifestyle, and environment. Personalized treatment plans will be based on data-driven insights, offering more effective interventions and reducing the trial-and-error approach that is often used in traditional medicine.
Improved Collaboration Between AI and Healthcare Professionals
Rather than replacing healthcare professionals, AI and ML will become essential tools that enhance the capabilities of doctors, dietitians, fitness coaches, and mental health professionals. These tools will help healthcare providers make better decisions by offering data-backed insights and recommendations, while still relying on human expertise for final judgment.
Greater Inclusivity and Accessibility
As technology becomes more affordable and accessible, AI-powered health solutions will become more inclusive. Lower-cost wearables and AI-powered apps will enable people from all walks of life to benefit from personalized health recommendations, making healthcare more equitable.
Conclusion
The future of personalized health, driven by AI and machine learning, promises to offer more efficient, customized, and accessible health solutions. Through AI-powered apps like Noom, Fitbit, and MyFitnessPal, individuals can receive highly personalized fitness, nutrition, and wellness recommendations based on their unique data. These technologies will continue to evolve, offering even greater insights and improvements in individual health outcomes. The integration of AI and ML into personalized health not only makes wellness more achievable but also empowers individuals to take charge of their health in a way that was once unimaginable.
The future of personalized health is undoubtedly intertwined with the continued evolution of AI and ML. These technologies have the potential to revolutionize how we approach fitness, nutrition, and overall wellness. However, significant challenges such as data privacy, algorithmic bias, accuracy, accessibility, and integration need to be addressed for AI-driven health solutions to reach their full potential.
As AI and ML continue to mature, their impact on personalized health will only grow, offering more precise, accessible, and effective healthcare solutions tailored to individuals’ unique needs and goals. With careful attention to overcoming these challenges, the future of personalized health powered by AI and ML is bright, promising a world where everyone has the opportunity to live healthier, longer lives.
SOURCES
Binns, M. (2020). AI and machine learning in healthcare: Challenges, trends, and opportunities. HealthTech Magazine.
Choi, S., & Kim, D. (2020). The role of artificial intelligence in personalized health care: Applications and future directions. Journal of Healthcare Engineering, 2020, 1–11.
Daoud, M., & Trabelsi, Z. (2019). Personalized health and fitness tracking with AI and machine learning technologies. International Journal of Computer Science and Information Security, 17(9), 101–108.
Gandomi, A., & Haider, Z. (2021). Big data analytics in healthcare: A review. Journal of Big Data, 8(1), 1–19.
Gupta, A., & Soni, D. (2021). Wearable technologies for healthcare: Trends, challenges, and opportunities. Journal of Medical Systems, 45(2), 1-13.
Li, S., Yang, Z., & Zhang, J. (2020). Machine learning-based predictive analytics in health care: A review. Healthcare Informatics Research, 26(1), 1–11.
Nundy, S., & Goldstein, L. (2020). Artificial intelligence and machine learning in health care: A review of the future. The Journal of Clinical Medicine, 9(7), 2301–2315.
Powell, J., & Isupova, M. (2021). The role of AI and data analytics in personalized wellness. Journal of Digital Health, 5(2), 21–30.
Shaban-Nejad, A., & Mihailidis, A. (2020). Artificial intelligence in healthcare: A review of the trends and challenges. Computers in Biology and Medicine, 123, 103838.
Toma, A., & Popescu, R. (2020). AI-based solutions for personalized health: Opportunities and challenges. International Journal of Environmental Research and Public Health, 17(6), 1943–1958.
Wen, S., & Liang, C. (2020). Exploring the role of artificial intelligence and machine learning in transforming healthcare systems. International Journal of Health Sciences and Research, 10(5), 108–116.
HISTORY
Current Version
December, 30, 2024
Written By
BARIRA MEHMOOD